Turing's Test
We cannot quite know what will happen if a machine exceeds our own intelligence, so we can't know if we'll be infinitely helped by it, or ignored by it and sidelined, or conceivably destroyed by it.
~Stephen Hawking
Important Concepts
Superintelligence
One thing that people are disappointed about when I talk to them about superintelligent AI is that I can't tell them how a superintelligent AI can harm them. But, by definition, a superintelligence's reasoning is beyond that of any human. We cannot know how or why a superintelligence might harm us. We don't even know if a superintelligence would want to harm us. The only aspect of superintelligence that we can speculate about is the process by which it will come about.1
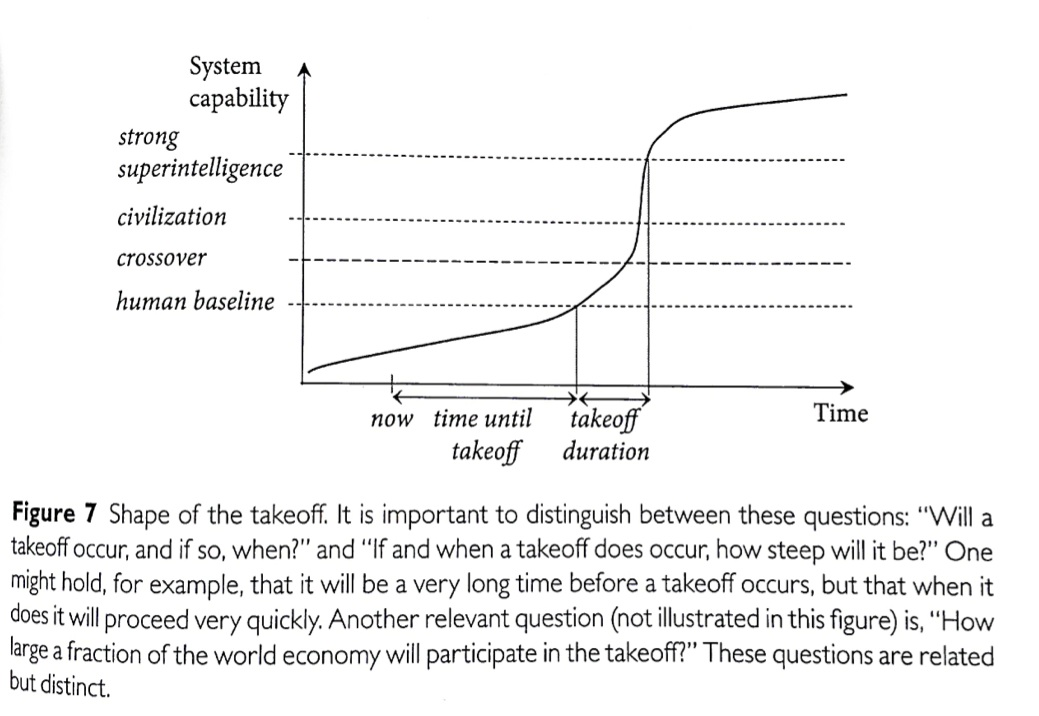
Diagram from Bostrom 2014:63.
A recurring topic in this area of research is that of an intelligence explosion. This occurs when machines finally surpass human-level intelligence. Call this point the singularity. Once the singularity has been reached, the machines themselves will be best equipped to program the next generation of machines. And that generation of machines will be more intelligent than the previous generation, and, again, will be best equipped to program the next generation of machines. After a few generations, machines will be much smarter than humans. And at a certain point, machine intelligence will rise exponentially, thus creating an irreversible intelligence explosion. This is one of the reasons why author James Barrat calls superintelligent AI our final invention (see Barrat 2013).
Routes to superintelligence
Bostrom details the multiple possible routes to machine superintelligence, some of which are detailed below. Bostrom also points out that the fact that there are multiple paths to machine superintelligence increases the likelihood that it will be reached...
Genetic algorithms
Genetic algorithms are algorithms that mimic Darwinian natural selection. Through this process, the "fittest" individuals ("fittest" being defined by whatever criteria the researcher desires) survive to "produce" offspring that will populate the next generation. It is already the case that natural selection has given rise to intelligent beings at least once. (Look in the mirror.) Although computationally intensive, this approach might serve to make minds again, albeit this time digital ones. Moreover, by applying machine learning to this process, we can expedite natural history. This is since machines might be intelligent enough to skip all the evolutionary missteps that nature might've taken. Before too long, we may be able to reach the singularity and an intelligence explosion will ensue.
AI seeds
This is an idea that Turing himself promoted. The goal here is not to create an intelligent adult mind, but rather a child-like mind that is capable of learning. Through an appropriate training period, researchers will be able to arrive at the adult mind. This will be faster than the training of humans, though, since the machine will not have natural human impediments (like the need for sleep, food, etc.). Once the machine reaches human-level intelligence, it is only a matter of time before the singularity is reached.
“Instead of trying to produce a programme to simulate the adult mind, why not rather try to produce one which simulates the child’s? If this were then subjected to an appropriate course of education, one would obtain the adult brain” (Turing 1950: 456).
Whole-brain emulation
Perhaps it is even possible to emulate human intelligence in a computer, without really knowing the basis of human intelligence. This could be done by first vitrifying a human brain (i.e., turning it into glass) and then sending in high-precision electron microscope beams to map out the entire neural architecture of the human brain. A sufficiently powerful computer will be able to process the data yielded from this method and emulate the functions of a human brain. Building off this, the singularity would be near indeed...
Why would any governments fund these kinds of research programs?
Bostrom reminds us that heads of state reliably enact programs that enable their nation to be competitive technologically, or, in some cases, to achieve technological supremacy. Our history is littered with examples. For example, China monopolized silk production from 3000 BCE to 300 CE. China also monopolized the production of porcelain (~600-1700 CE). More recently, the USA achieved military supremacy (or something close to it) with its atomic weaponry (1945-1949).
Relatedly, we have reason to believe that a war between the Great Powers will occur in the 21st century. In Destined for War, Graham Allison argues that in most historical cases where there is an established hegemonic power (e.g., U.S.A.) and a rising competitor (e.g., China) the result is war. In Climate Wars, Gwynne Dyer details the existing war plans of the major powers in the case that the effects of climate change begin to take place, thereby rendering certain geographical regions agriculturally barren and displacing entire populations. As you can see, there is a real threat of war in the near future. Moreover, as you will see later in this lesson, artificial intelligence would be an invaluable asset to have during conflict. It's also the case that artificial intelligence may converge with nanotechnology, which would almost ensure that the possessor of these technologies would have absolute military supremacy (if not a global dictatorship). So the answer is simple: governments invest in AI because other governments do. The race for mastery over AI may be the race for the mastery of war.
Our only hope...(?)
The potential threat of this line of research necessitates that we devote resources to answering questions surrounding the possibility of superintelligence. One of the main problems, of course, is figuring out a way to know if the singularity has been reached. But prior to this, we must figure out how to know if a computer is intelligent at all. Is the Turing test our best bet? Unlikely. In fact, it's already been passed.
Clearly we need a better test...
Atoms controlled
by superintelligence...
The end...
What is to be done?
Preparing for an intelligence explosion...
In his chapter of Brockman's (2020) Possible Minds, Estonian computer programmer Jaan Tallinn reminds us that the dismissal of the threat of AI would be ridiculous in any other domain. For example, if you’re on a plane, and you’re told that 40% of experts believe there’s a bomb on that plane, would you wait around until the other 60% agreed or would you get off the plane? Many share this alarm that Tallinn expresses over the lack of political will to invest more time and energy on safety engineering in AI. In his chapter of the same volume, physicist and machine learning expert Max Tegmark dismisses the accusations that this is fear mongering. Just like NASA thought out every thing that might go wrong during its missions to the moon, he argues, the risk analysis of AI is simply the basic safety engineering that must be performed on all technologies.
Having said that, our political system looks to be highly dysfunctional and certainly not capable of mustering the political will to begin to legislate on AI safety engineering. Moreover, elected officials are unlikely to be knowledgeable in the relevant science to intelligently debate the issues—apparently the average age in the Senate a few years ago was 61 years old. And even if they were up-to-date on machine learning, the gridlock of the two-party system would block progress. In fact, some (including Los Angeles mayor Eric Garcetti) think that political parties are doing more harm than good.2
The idea that the American political system is not poised to address complicated issues is nothing new. Physicist Richard Feynman (1918-1988), who took part in the building of the atomic bomb as part of the Manhattan Project and who taught at CalTech for many years, had this to say about what he called our "unscientific age":
"Suppose two politicians are running for president, and one goes through the farm section and is asked, 'What are you going to do about the farm question?'' And he knows right away—bang, bang, bang. [Then they ask] the next campaigner who comes through. 'What are you going to do on the farm problem?' 'Well, I don't know. I used to be a general, and I don't know anything about farming. But it seems to me it must be a very difficult problem, because for twelve, fifteen, twenty years people have been struggling with it, and people say that they know how to solve the farm problem. And it must be a hard problem. So the way I intend to solve the farm problem is to gather around me a lot of people who know something about it, to look at all the experience that we have had with this problem before, to take a certain amount of time at it, and then to come to some conclusion in a reasonable way about it. Now, I can't tell you ahead of time the solution, but I can give you some of the principles I'll try to use—not to make things difficult for individual farmers, if there are any special problems we will have to have some way to take care of them,' etc., etc., etc. Now such a man would never get anywhere in this country, I think... This is in the attitude of mind of the populace, that they have to have an answer and that a man who gives an answer is better than a man who gives no answer, when the real fact of the matter is, in most cases, it is the other way around. And the result of this of course is that the politician must give an answer. And the result of this is that political promises can never be kept... The result of that is that nobody believes campaign promises. And the result of that is a general disparaging of politics, a general lack of respect for the people who are trying to solve problems, and so forth... It's all generated, maybe, by the fact that the attitude of the populace is to try to find the answer instead of trying to find a man who has a way of getting at the answer" (Richard Feynman, Lecture III of The Meaning of It All).
Automation
Regardless of whether there is ever an intelligence explosion, it is certainly the case that automation will continue. As stated in the Food for Thought in the last lesson, some possible solutions include a universal basic income, Kai-fu Lee's social investment stipend, and even Milton Friedman's negative income tax—the latter of which is a conservative proposal (although there is some conservative support for a universal basic income too). Only time will tell if our political system will be able to pass any legislation that moves in any of these directions.
García's Two Cents
My own views are not the point of this lesson or this course, so I'll keep my comments brief here. I've been making the case, for several years now, that one direction we ought to move towards is that of expanding the information-processing power of the population. We should, I believe, boost human intelligence as far as is possible. For example, we should ensure the population is well-nourished, we should eliminate neurotoxins (such as lead), and we should engineer safe and effective nootropics (substances that enhance cognitive functioning). At the very least we should make college free for all. The idea here is not linked to socialism or any partisan framework. It is simply the case, I believe, that problems like global climate change, the threat of AI, and others will only be solved through human intelligence. And so, we should expand the catch-net of human talent. Currently, too many minds are not being given the chance to contribute because they are dealing with issues that, I think, a rich industrial country should have ameliorated by now: lack of housing, food insecurity, environmental toxins, racial and gendered prejudice, etc.3
<
Early in the history of the field of artificial intelligence, the dominant paradigm was the physical symbol systems hypothesis (PSSH). In a nutshell, this is the assumption that many or all aspects of intelligence can be achieved by the manipulation of symbols according to strictly defined rules, an approach to programming that rigorously specifies the procedure that a machine must take in order to accomplish some task.
In the 1980s, PSSH was replaced by connectionism as the dominant paradigm in AI research. Connectionism is the assumption that intelligence is achieved via neural networks—whether they be artificial or biological—where information is stored non-symbolically in the weights, or connection strengths, between the units of the neural network. In this approach, a machine is allowed to "update itself" (i.e., update the connection strengths between its neurons) so as to improve at some task throughout a training period, much like human brains rewire themselves during learning.
Machine Learning (ML), which utilizes neural networks, has been the dominant method in AI since around 2000, with deep learning being the dominant form of ML since about 2012. ML has led to breakthroughs in the field of AI, primarily with regards to narrowly-defined tasks. Nonetheless, these breakthroughs are accumulating such that many tasks and jobs that humans used to perform are now being automated. We also covered four possible futures that further progress in AI might bring about: a loss of meaning in human activities, a jobless economy, the merging of AI and nanotechnology for nefarious ends, and "unfriendly" AI.
As of now, there is still a dispute about what mental states are, what mental processes are, and what constitutes intelligence. The hard problem of consciousness is far from solved, there is little political will to solve large-scale existential risks for humanity, and the clock is ticking.
FYI
Supplemental Material—
-
Video: TEDTalks, How civilization could destroy itself -- and 4 ways we could prevent it | Nick Bostrom
Related Material—
- Video: Financial Times, US scientists prepare for high-tech wars of the future
- Video: TEDTalk, How AI can save our humanity | Kai-Fu Lee
Advanced Material—
-
Reading: Nick Bostrom, The Vulnerable World Hypothesis
Footnotes
1. In chapter 7 of Superintelligence, Bostrom gives some views about the relationship between intelligence and motivational states in superintelligent AI. He first defines intelligence as flexible but efficient instrumental reasoning, much like the definition that we used last time of an AI being an optimization process in general. With this definition in place, if one subscribes to the view that basically any level of intelligence can be paired with basically any final goal, then one subscribes to the orthogonality thesis. This means that one cannot expect a superintelligence to automatically value, say, wisdom, renunciation, or wealth. The orthogonality thesis pairs well with the instrumental convergence thesis, the view that any general intelligence might arrive at roughly the same set of instrumental goals while attempting to achieve their final goals. These instrumental goals might include self-preservation, enhancing one’s own cognition, improving technology, and resource acquisition. Personally, I reject the orthogonality thesis. There appears to be a necessary connection between the system that produces motivational states and the system that makes inferences (see Damasio 2005). In particular, it appears that the system that makes motivational states performs a dimensionality reduction on the possible inferences that can be made—an inference strategy that is known as bounded optimality. And so, it is unlikely that any motivational system can be paired with any degree of intelligence. An intelligence equivalent to or greater than that of humans must in some way resemble human cognition, since it needs a motivational system that performs a dimensionality reduction. Absent this, it is not really an intelligence but a data cruncher. Data crunching, however, is not intelligence, even at the scale of a supercomputer. As Bostrom admits, intelligence is efficient. This is the case in humans (Haier 2016). It seems fair to assume that real machine intelligence will face a similar requirement.
2. The interested student can check out this episode of Freakonomics, in which the idea that the two dominant political parties are essentially a duopoly that is strangling the American political system is explored.
3. For the sake of fairness, I should add two criticisms of my view here, for those that are interested. First, one criticism of my view is that boosting the population's information-processing power might actually lead to the aforementioned intelligence explosion, and so my solution actually leads to the problem I'm trying to solve. I don't think this is a good argument, but it has been made against my view. Second, as far as I know, there is no name for my set of political beliefs. I sometimes joke and call myself a neo-prudentist—a made-up political view. However, some friends and colleagues disparagingly (but lovingly) refer to my views as a form of techno-utopianism, somewhat like that of Francis Bacon.